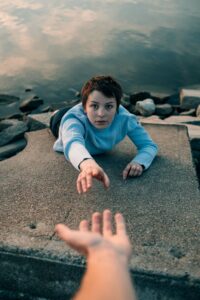
Trust and uncertainty
We live in a world with significant health, economic, climate, human population and geopolitical pressures. At the same time, we have rapid advances in technology, especially digital and AI, that are transforming our work, play and finances.
In general, we hope that advances in technology will help us to solve the significant challenges of our age. But the transformation still has a long way to go.
In the early days of the Internet and e-commerce, traditional “bricks and mortar” businesses tried to resist change and new technology. Even so, we now have Amazon and other digital, Internet based platforms dominating the scene. These are the largest, most successful companies today.
Commensurate with the rapid growth in computers, computing power, Internet, Internet of Things (IoT), we have seen this explosion in data and big data. Out of this, we are seeing the emergence of probably the most transformative and pervasive technology yet, Artificial Intelligence (AI). And yes, Amazon also is a leader in the application of AI.1
AI depends on accurate, timely, reliable computer models of processes and the environment it operates in. But, using our measurements, concepts and models to analyse and control processes will always result in errors and uncertainty, because the models are not reality. They are only approximations. And the very act of observing and measuring a particle or a conscious being, can alter its behaviour – more on this below.
Trust in our AI models is of paramount importance for the more widespread acceptance of AI in industry, health care and society. Based on a recent review, this discussion highlights some of the trust issues.
From a review carried out by PCA, three key issues or opportunities have been highlighted:
Trust
Sustainability
Digitisation
These are interrelated and they all have something in common, uncertainty.
These issues and opportunities existed before the global pandemic, and have now been accelerated by it.
So, how is trust, sustainability and uncertainty being addressed in Artificial Intelligence (AI)?
AI Models and Reality
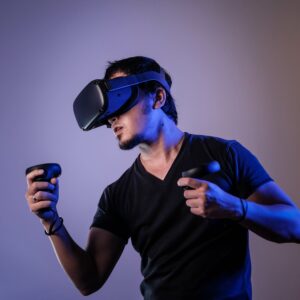
AI models, virtual reality and reality
Some AI models are using combinations of physical, natural science, laws and statistical modelling to describe processes. Reference the recent work at University of Delaware.2
These types of hybrid models could be more reliable than models based only on statistics, because they build in understanding of the process. But we need to be aware that the so called “laws of nature” are actually laws discovered by people. We have developed these laws to describe and explain observed regularities in this world and the extended universe. Even so, people are aspects of nature too, so maybe conscious, intelligent life is nature’s way of discovering itself!
In the natural sciences, mathematics and statistics, we use observations, concepts, thoughts, symbols, words, measurements, calculus to model the regularities of the world around us. These use the analytical mind to break down reality into bits based on our defined units of measurement. Measurement units are abstract conventions created by humans, they do not somehow exist out there in reality, but rather have been added to reality by us. Observation and measurement has provided us with very powerful tools to model our world.
Using our measurements to divide reality into bits is a powerful tool for description and quantification, but we also know that everything is energy in some form or other. So, reality is more like continuous, pulsating energy waves over vast ranges of scale. For example, electromagnetic waves (light, radio, etc.) range from ultra high frequencies measured in nano metres to extremely long wave lengths measured in meters. And, there are infinite numbers of frequencies in-between any two frequencies, because in reality it is a continuous range, not actually divided into bits.
Then, on much larger scales, we have the energy cycles of life, companies, industries, societies, economies, planets, stars, galaxies, the universe itself. Let’s not even go there!
Just like God, no one really knows what reality is. We have images and concepts, but that is all they are.3
Some believe that the universe and life is random and that we are all insignificant statistical flukes in a mechanistic universe. Others, including most philosophies and theologies, assert that there is an underlying reality or continuum to everything. And some say the universe is more like an intelligent organic system, where the intelligence of the whole is greater than its parts, so called synergy.4 Indeed, scientists are now observing remarkable similarities between the structure of the brain and the universe itself, but, of course, on vastly different scales!5
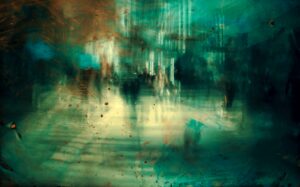
What is reality?
What we do know is that reality consists of these energies in various forms. Continuous, wiggly, waves of energy vibrations over wide ranges of frequency spectrums. Something like a “dance” of fundamental positive and negative forces. When the environmental conditions are right, this “dance” can sometimes lead to complexity, consciousness and intelligence. Matter, including us, is energy. The universe and environment inside and outside us is a network of relationships, a relativity system all contained by and ordered by space. We mostly ignore space because it is transparent, colourless. But space is fundamental, because it contains and connects all of life, planets, stars, galaxies, everything!
Therefore, all our models that divide reality into bits, separate things and events, including human cognition (the models we have in our minds) and AI, are approximations to reality, not actually reality. AI models are certainly powerful tools, but they are not infallible. We need to be aware of the limitations and risks of AI. Being open about it and having the necessary safeguards built into the models is essential to build trust.
Sustainability Consciousness and AI (The Future?)
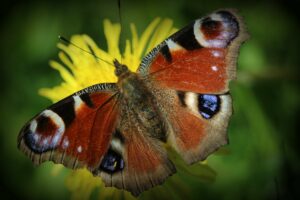
The organism-environment
Biologists and ecologists now know that humans and other organisms do not exist separately from their environment, but are mutually interdependent systems, better described as the organism-environment.6 Species live off each other and also cooperate in symbiotic relationships.7 The science has given credibility to our feelings about the nature of the world.
This realisation has permeated our collective consciousness, because there are now increasing stakeholder demands on governments, industry and society regarding sustainability.8
It also implies that, in order to build trust in AI, we need to build eco-sustainability into our AI systems and, the awareness of eco-sustainability.
There are examples where AI is being used to reduce emissions in industry and other sustainability goals.9 But, a report by PwC UK, commissioned by Microsoft, “How AI can enable a sustainable future”10, states “a current lack of awareness, engagement and prioritisation: governments, companies, academics and investors are not currently focused on, or prioritising, AI for environmental applications”. Against this, it explains there are significant opportunities for AI to improve the environment, economy and productivity.
By aligning AI with sustainability, an important spin off could be that trust in AI itself is improved. If that happens, people can clearly see that AI is helping to meet the sustainability objectives of society as a whole.
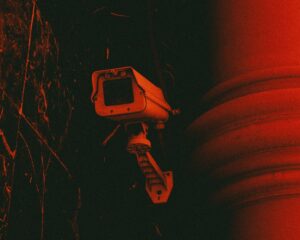
Mass surveillance with AI
Rather than the current fears that it is more about military applications, mass surveillance by governments, facial recognition, population control and job losses.
An AI that works to protect our environment for organic life is surely more trustworthy than an AI that does not. And, we can trust it even more, if the AI itself also needs an healthy environment for its own survival. Otherwise, it is possible to conceive of a future where AI has wiped out all life and our environment, leaving only AI machines on the planet!
Perhaps we need a more organic form of AI that also depends on our environment for its own sustainable existence. Then, the life objectives of humans and AI will surely be more compatible with each other.
We are probably decades away from that sort of advanced stage of AI, but we should take renowned scientist, Stephen Hawking’s warnings seriously. Stephen Hawking wrote his final warning for humanity in his book, Brief Answers To The Big Questions11. His main warning was about AI. As Hawking puts it, “Whereas the short-term impact of AI depends on who controls it, the long-term impact depends on whether it can be controlled at all.”
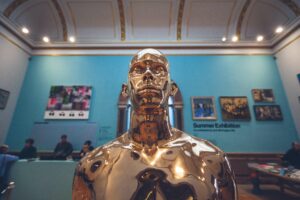
Super human elite?
Hawking also warned that wealthy elites will use genetics to edit genes for intelligence, memory and lifespan, so called “self-designed evolution.” He predicted that in this century, they will be able to develop into superhumans, outcompeting everyone else. The other warning is that climate change will eventually destroy the environment. The superhumans will inhabit other planets, leaving the rest of us behind to die out!
Science fiction writer, Isaac Asimov wrote his Three Laws of Robotics as a way to stop AI from hurting people. Today’s AI developers realise that these laws won’t stop AI from potentially harming us. Instead, one approach is to develop AI that is empowered to pick the best scenario for any given situation.12
It is this author’s opinion that, in addition to AI empowerment, eco-sustainability should be encoded into all AI models of how the world works. So long as the AI’s model of the world includes eco-sustainability, an empowered AI should act not to harm itself, humans or our shared environment. This should be even more true, if the AI itself is manufactured to depend on biodiversity, clean air, clean water, clean land and clean energy for it’s very existence. An organic AI, just like humans and all life on Earth!
In fact, as we are no doubt already aware, this is already happening and is not just science fiction. Work at the US Defense Advanced Research Projects Agency (DARPA) was described by former DARPA director Arati Prabhkar in an article, “The merging of humans and machines is happening now”.13
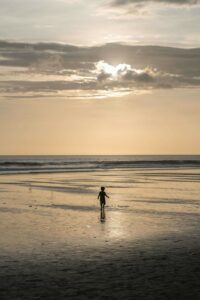
An eco-sustainable future for our children?
This article was written in 2017. Somewhat disappointingly, it does not mention the importance of building eco-sustainability into the AI. Even so, the augmentation of humans with AI capabilities is actively being developed in medical, military and some other applications. As Prabhkar says, “We and our technological creations are poised to embark on what is sure to be a strange and deeply commingled evolutionary path”.
It’s probably a good thing that organic AI seems to be our future trajectory, because the existence of this type of AI depends on eco-sustainability and maintaining an healthy environment.
But we still need to beware of the non-human AI machines and the need for eco-sustainability in AI. That way eco-sustainability will win and everyone can benefit.14
It also means we can expect to have superhumans this century, based not only on genetic modification, but also on AI augmentation. As Stephen Hawking warned, the danger is that these technologies will preferentially go to the elite, who will increasingly outcompete everyone else.
As we tamper with the very structure of life itself, we have to ask whether we have the wisdom to know all the possible consequences, including the possibility of hostile actions such as cyber attacks.15
As an example of how the future is closer than we think, Google DeepMind’s AlphaFold has just reported the ability to solve the 50 year old grand challenge of biology, solving the “protein folding problem”.16 This is the 3-dimensional shape that different proteins exhibit in nature and which is important for how they function. This breakthrough in understanding demonstrates the impact AI can have on scientific discovery and its potential to dramatically accelerate progress in some of the most fundamental fields that explain and shape our world.
Humanity and Human Values
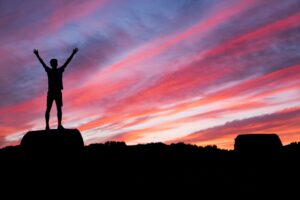
We know that reality is energy
Human conscious attention is a linear scanning system, using the senses of vision, hearing, feeling, taste, smell. It is only aware of a very limited range of these energy spectrums and relationships around us. Even with our sensitive scientific instruments, we have limitations. Since humans have limited conscious attention, it follows that our AI models also will have these limitations of conscious attention. In the case of AI, the conscious attention is based on the particular linear scanning capabilities of the sensors used to send signals to the AI’s “brain”.
These limitations of sensing and monitoring the world around us are part of what makes us human. It’s as if we know the world is not clear cut, that there is always uncertainty and we do not know it all. So long as we are healthy, most of the time, we are not even conscious of our own bodies and brain. For example, you don’t have to know how your body works, eyes see, brain thinks, heart beats, hair grows, etc. All these amazing physiological processes just happen without you having to know how!17 18
We should be aware that the limitations of conscious attention also can result in selection bias. This is where we base our models on data that has been selected to represent a population or process, but the data has not been properly randomised. In those cases, the sample data is not representative of the population or process being studied and modelled, possibly leading to incorrect opinions, conclusions and actions.19 20
And here is another important consideration. Is a person more trustworthy when she believes that absolute laws apply? Absolutes that command you must absolutely not do this or that! Such a person or AI could be more dangerous, because they put rigid structures above fair play or equity. A good judge should have a sense of equity or use a rule of thumb, because every case is different and some latitude is needed. A person or AI who holds to absolute rules will be inflexible when it comes to the test. These qualities are not easily learned from a book. They are more experiential.
It is a challenge to build these human qualities into our AI. So, the risk is that the AI will take its programmed laws absolutely seriously and become too inflexible – too inhuman. The art of life is to have a certain rigidity but always know when to give, come off your high horse when necessary. Without this flexibility, life becomes a contest where you have to win at any cost. And this can lead to dangerous situations, especially when critical decisions are being made!
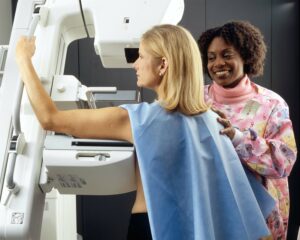
Critical decisions require the best human qualities
People or AI who take things too seriously can be inflexible, unable to back down. The thought that they have to win can make them nervous and they start worrying. Anxiety, indecision, excessive feedback, poor decisions and accidents can be the result.
So, this attitude of rigidity, of always adhering to absolute laws can be a waste of energy and unsustainable in the long run.
The qualities of fair play, humour, judgement, humility are what make us human and trustworthy. Probably the most valuable human trait, is the ability to not take things too seriously. To be able to judge when to de-escalate situations.
Somehow our AI needs to have these qualities. If this is difficult to achieve, it needs to know when the uncertainty is too high for it to take certain actions. In those situations, it should defer to a second opinion (preferably more than one human) for safety, sustainability or financial reasons.21 22
Trust and ethics are so important that the EU has adopted a set of Ethics Guidelines for Trustworthy AI.23 Based on fundamental rights and ethical principles, the Guidelines list seven key requirements that AI systems should meet in order to be trustworthy:
- Human agency and oversight.
- Technical robustness and safety.
- Privacy and data governance.
- Transparency.
- Diversity, non-discrimination, and fairness.
- Environmental and societal wellbeing.
- Accountability.
Changing Environments, Multiple Factors, Causation
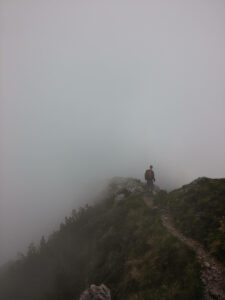
Changing environment and uncertainty
We live in a world with a complex network of interrelationships. It can have thousands of variables operating at any single point. Some variables will be more dominant at any given time, but if the environment changes, other variables can become more influential. The behaviour of the process will then be different in the new environment.
Again, this has implications for AI models that are highly tuned to a given environment. As in machine learning, where the model is trained on historical data sets. But, when the environment changes, the model needs to adapt to the new environment. It may be able to cope with small changes within certain limits, but large changes, especially random, unexpected events, are a different matter altogether.
The behaviour of a particle, process or organism depends on its context or relationships; when is it, where is it? In other words, it’s behaviour depends on the environment it is in. For example, the behaviour of blood inside a test tube will be different to its behaviour in the living organism. The behaviour
of a person may be different, depending on where they are and who they are with (different social and cultural norms).
When analysing situations and processes, we need to appreciate that assigning definite causes is not a simple thing to do. Causation is useful only when the cause is a single factor, and the connection is clear.24 Even then, are the cause and effect really separate events or, are they really one connected, energy pattern that can repeat? Much like the organism-environment that we discussed earlier.
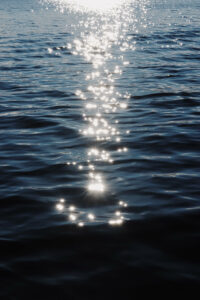
Continuous energy, waves, reflections
It is not commonly known that in language, future events can influence earlier events. How so? For example, read the following two sentences and notice how the meaning of the word “bark” is altered by the later (future) part of the sentence:
The bark of a tree.
The bark of a dog.
And we know that humans frequently make decisions in the present based on what we think will happen in the future. Is that the present influencing the future or, an imagined, virtual future influencing the present? In the world of physics and quantum mechanics, what you do today could affect what happened yesterday!25
So, when you look at it more deeply, things are not always what they seem on the surface. Cause and effect can be complicated in this multi factor world. Complex, interdependent multifactor causes arise often in nature, science and industry. And cause and effect events can be viewed as connected patterns of energy. Continuous interconnected processes, rather than isolated, separate events.
Machine Learning
Machine learning is the basis of AI models. In general, machine learning uses historical data sets and statistics to learn and build models. This means someone needs to select the best historical data sets for the machine to learn from. And this can require considerable judgement. It also means that the machine AI models will always be a reflection of the past. But past performance is not always what you want. If it is what you want and you are able to use good judgement in selecting the best data sets to train the machine, a machine AI model can be useful for automation tasks.
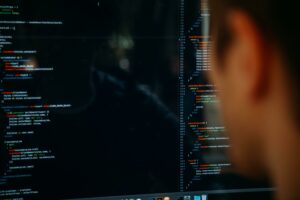
Machine learning algorithms
Deep learning is a more advanced AI. It is a complex development of machine learning and AI that uses layered structures of algorithms called artificial neural networks (ANN). The layered algorithms are interconnected somewhat like the neurones of the brain. The aim is for it to learn from the data it is given and make its own intelligent decisions. Examples are speech recognition systems such as Google Assistant and Amazon Alexa. Also, robots and self-driving cars.26 27
However, deep learning is very data and compute resource intensive, because huge numbers of parameters are needed for neural net models to be accurate. This is now raising questions about energy efficiency and sustainability, for example, the risks of large language models. It also takes a long time to develop and train an accurate model, hours or even months. Regarding trust and uncertainty, it is difficult to know how they come to particular conclusions (black box syndrome)28 and, they may not cope well with changing environments. For example, a neural net trained to drive a car on sunny days could fail on rainy days.
There are other types of machine learning algorithms. According to the “No Free Lunch” theorem of AI, there is no perfect algorithm that works equally well for all tasks. It follows that different problems can require different algorithmic tools.29
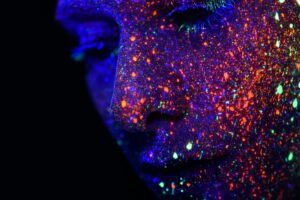
AI in the machine
In supervised learning, humans provide clear guidelines to train the machine how to use certain data sets with certain algorithms.30 With unsupervised learning, the machine is allowed to use certain algorithms itself to search for patterns in the data sets (clustering or classifying the data on its own). Computers can be very good at finding patterns in data that humans might miss.
Semi-supervised learning is where the programmer combines labelled data (data correctly identified as being a certain type or category) with unlabelled data, then allows the machine to decipher the unlabelled data itself. Unlabelled data is plentiful, but labelled data more expensive to source.31
Reinforcement learning is the latest cutting-edge that holds promise in making machines creative. It is where the machine learns through trial and error in dynamic environments, not only with static historical data sets. Somewhat similar to the way humans learn through positive and negative reward signals based on our actions.32
In the case of deep reinforcement learning, a neural network stores the experiences to improve the way the task is performed. For example, the success of Google DeepMind’s AlphaGo and AlphaFold.33 34 35 36
Reinforcement learning, deep learning, and machine learning each have their place and specific application areas. No one of them is going to replace any of the others.
Risk Management in AI Implementation
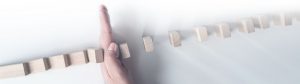
PCA is available to provide independent audits and increase trust in AI
A recent survey by Deloitte found that risk concerns are holding back AI adoption.37 38
Research suggests that actively managing AI risk boosts the technologies’ benefits to the organisation. In the survey, the leading risk management organisations undertake more than three AI risk management practices and align their AI risk management with their organisation’s broader risk management efforts.
These Leaders believe AI has greater strategic importance to their business: 40% see AI as “critically important” to their business today.
Compared to the majority of organisations, who are only dabbling in AI, these leaders consider it to be more strategically important.
Deloitte make the following suggestion, “For their part, AI solution providers may be able to improve their competitive positioning by incorporating risk management into their offerings. We suggest sharpening your risk management game: Certify that you perform regular auditing and testing of your AI systems to help ensure accuracy, regulatory compliance, and lack of bias. By reducing risks for your customers, you can be better positioned to build customer trust”.39 40 41
Here at PCA, we agree with Deloitte. The PCA service is part of the digital ecosystem. It is available to provide independent audits and increase trust in AI.
For more examples of how AI, sustainability and other technology trends are developing around the world, follow us on our new evolving aggregator feed on Twitter. It is based on data rules, interests, defined by PCA cognition. From science, industry, tech, philosophy, business and other sources. Keep up with the technology trends, innovations and risks that could assist or disrupt your business.
Sources
- How Amazon Leverages Artificial Intelligence to Optimize Delivery, 22 Oct 2019, Catie Grasso https://feedvisor.com/resources/amazon-shipping-fba/how-amazon-leverages-artificial-intelligence-to-optimize-delivery/
- Successfully Evaluating AI Models in Industry, 21 Oct 2020
https://process-chemistry-analytics.com/index.php/2020/10/21/successfully-evaluating-ai-models-in-industry/ - The Consciousness of Reality, 11 Apr 2019, James B. Glattfelder
https://link.springer.com/chapter/10.1007/978-3-030-03633-1_14 - SYNERGETICS, Buckminster Fuller Institute
https://www.bfi.org/about-fuller/big-ideas/synergetics - Study Maps The Odd Structural Similarities Between The Human Brain And The Universe, 17 Nov 2020, Michelle Starr
https://www.sciencealert.com/wildly-fun-new-paper-compares-the-human-brain-to-the-structure-of-the-universe - The theory of the organism-environment system: I. Description of the theory, Dec 1998, Järvilehto T.
https://pubmed.ncbi.nlm.nih.gov/10333975/ - Malmstrom, C. (2010) Ecologists Study the Interactions of Organisms and Their Environment. Nature Education Knowledge 3(10):88
https://www.nature.com/scitable/knowledge/library/ecologists-study-the-interactions-of-organisms-and-13235586/ - 10 Reasons Sustainability Needs To Be Part Of Your Digital Transformation Strategy, 8 Nov 2020, Louis Columbus
https://www.forbes.com/sites/louiscolumbus/2020/11/08/10-reasons-sustainability-needs-to-be-part-of-your-digital-transformation-strategy - ‘Tech for Good’: Using technology to smooth disruption and improve well-being, 15 May, 2019 | Discussion Paper, McKinsey Global Institute
https://www.mckinsey.com/featured-insights/future-of-work/tech-for-good-using-technology-to-smooth-disruption-and-improve-well-being - How AI can enable a sustainable future – Estimating the economic and emissions impact of AI adoption in agriculture, water, energy and transport. 2020, PwC UK, report commissioned by Microsoft.
https://www.pwc.co.uk/services/sustainability-climate-change/insights/how-ai-future-can-enable-sustainable-future.html - Stephen Hawking’s final warning for humanity: AI is coming for us, 16 Oct 2018, Abigail Higgins
https://www.vox.com/future-perfect/2018/10/16/17978596/stephen-hawking-ai-climate-change-robots-future-universe-earth - Asimov’s Laws Won’t Stop Robots from Harming Humans, So We’ve Developed a Better Solution, 11 Jul 2017, Christoph Salge, Scientific American
https://www.scientificamerican.com/article/asimovs-laws-wont-stop-robots-from-harming-humans-so-weve-developed-a-better-solution - The merging of humans and machines is happening now, 27 Jan 2017, Arati Prabhakar, DARPA
https://www.wired.co.uk/article/darpa-arati-prabhakar-humans-machines - We read the paper that forced Timnit Gebru out of Google. Here’s what it says. The company’s star ethics researcher highlighted the risks of large language models, which are key to Google’s business. 4 December 2020, Karen Hao, MIT Technology Review
https://www.technologyreview.com/2020/12/04/1013294/google-ai-ethics-research-paper-forced-out-timnit-gebru - This new cyberattack can dupe DNA scientists into creating dangerous viruses and toxins, 30 Nov 2020, Charlie Osborne
https://www.zdnet.com/article/this-new-cyberattack-can-dupe-scientists-into-creating-dangerous-viruses-toxins - AlphaFold: a solution to a 50-year-old grand challenge in biology, 30 Nov 2020, The AlphaFold team, Google Deep Mind
https://deepmind.com/blog/article/alphafold-a-solution-to-a-50-year-old-grand-challenge-in-biology - Not What Should Be – Pt. 1, Alan Watts
https://www.alanwatts.org/1-1-1-not-what-should-be-pt-1/ - The Consciousness of Reality, 11 Apr 2019, James B. Glattfelder
https://link.springer.com/chapter/10.1007/978-3-030-03633-1_14 - Catalogue of Bias Collaboration, Nunan D, Bankhead C, Aronson JK. Selection bias. Catalogue Of Bias 2017:
http://www.catalogofbias.org/biases/selection-bias/ - We read the paper that forced Timnit Gebru out of Google. Here’s what it says. The company’s star ethics researcher highlighted the risks of large language models, which are key to Google’s business. 4 December 2020, Karen Hao, MIT Technology Review
https://www.technologyreview.com/2020/12/04/1013294/google-ai-ethics-research-paper-forced-out-timnit-gebru - A neural network learns when it should not be trusted, 19 Nov 2020, Daniel Ackerman, Massachusetts Institute of Technology
https://techxplore.com/news/2020-11-neural-network.html - The Road to Responsible AI – Avoiding instrumental convergence with uncertainty, Nov 2020, Greg Chapman
https://medium.com/swlh/the-road-to-responsible-ai-ed1d7ccebb86 - Ethics Guidelines for Trustworthy AI, Apr 2019, European Commission
https://ec.europa.eu/futurium/en/ai-alliance-consultation/guidelines - The Slippery Math of Causation, 30 May 2018, Pradeep Mutalik
https://www.quantamagazine.org/the-math-of-causation-puzzle-20180530/ - Can the future affect the past? 3 Aug 2012, Philip Ball
https://physicsworld.com/a/can-the-future-affect-the-past/ - What is a Neural Network | Neural Networks Explained in 7 Minutes | Edureka, 30 Aug 2019
https://youtu.be/vpOLiDyhNUA - Neural Network Architectures and Deep Learning, 5 Jun 2019, Steve Brunton, Uni. of Washington
https://youtu.be/oJNHXPs0XDk - Practical Explainable AI: Unlocking the black-box and building trustworthy AI systems, 4 July 2020, Raheel Ahmad
https://www.aitimejournal.com/@raheel.ahmad/practical-explainable-ai-unlocking-the-black-box-and-building-trustworthy-ai-systems-2 - There is No Free Lunch in Data Science, Sep 2019, Sydney Firmin, KDnuggets (19:n35)
https://www.kdnuggets.com/2019/09/no-free-lunch-data-science.html - Supervised Learning, 19 Aug 2020, IBM Cloud Education
https://www.ibm.com/cloud/learn/supervised-learning - Hady M.F.A., Schwenker F. (2013) Semi-supervised Learning. In: Bianchini M., Maggini M., Jain L. (eds) Handbook on Neural Information Processing. Intelligent Systems Reference Library, vol 49. Springer, Berlin, Heidelberg. https://doi.org/10.1007/978-3-642-36657-4_7
- 5 Things You Need to Know about Reinforcement Learning, Mar 2018, Shweta Bhatt, KDnuggets (18:n14)
https://www.kdnuggets.com/2018/03/5-things-reinforcement-learning.html - What is reinforcement learning? The complete guide, 5 July 2018, Błażej Osiński and Konrad Budek
https://deepsense.ai/what-is-reinforcement-learning-the-complete-guide/ - Is AI finally closing in on human intelligence? 13 Nov 2020, John Thornhill, The Straits Times
https://www.pressreader.com/singapore/the-straits-times/20201113/281878710903552 - Deep Reinforcement Learning: Pong from Pixels, 31 May 2016, Andrej Karpathy blog
http://karpathy.github.io/2016/05/31/rl/ - An introduction to Reinforcement Learning, 2 Apr 2018, Arxiv Insights
https://youtu.be/JgvyzIkgxF0 - Getting ahead of the risks of artificial intelligence – Leading AI adopters manage potential risks and achieve better outcomes, 19 Nov 2020, Susanne Hupfer, Deloitte
https://www2.deloitte.com/us/en/insights/industry/technology/risks-of-artificial-intelligence.html - Reality check: Analysts check in on the AI hype cycle – AI applications still come with significant hype, but with a targeted approach, organizations can get the most out of their applications, 23 Nov 2020, Joseph M. Carew
https://searchenterpriseai.techtarget.com/feature/Reality-check-Analysts-check-in-on-the-AI-hype-cycle - These new metrics help grade AI models’ trustworthiness, 2 Dec 2020, Ben Dickson
https://thenextweb.com/neural/2020/12/02/these-new-metrics-help-grade-ai-models-trustworthiness-syndication/ - CAN AI REALLY KNOW WHEN IT SHOULDN’T BE TRUSTED, 30 November 2020
https://mindmatters.ai/2020/11/can-ai-really-know-when-it-shouldnt-be-trusted/ - Derisking AI by design: How to build risk management into AI development, 3 Dec 2020, Juan Aristi Baquero, Roger Burkhardt, Arvind Govindarajan and Thomas Wallace, McKinsey & Co
https://informaconnect.com/derisking-ai-by-design-how-to-build-risk-management-into-ai-development/